June 1, 2023 | AI and NLP, Quality Improvement, Year Round Prospective HEDIS®
Tackling Prospective HEDIS® Review – Tips and Tricks
Many payers are either experimenting with or fully implementing a prospective HEDIS® review process. If you’re looking for strategies to optimize your prospective review, these ten tips and tricks will help you derive maximal value.
Many payers are either experimenting with or fully implementing a prospective HEDIS® review process. This process uses medical record review (MRR) to close gaps during the measurement year and on a much larger population than the HEDIS® sample (potentially your entire population) during the measurement year. The use of clinical data is associated with higher quality rates and better management of populations. Using clinical data is especially important as the hybrid measures are removed, leaving payers potentially vulnerable to rapid rate drops and lost incentives.
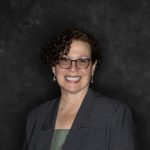
Rebecca Jacobson, MD, MS, FACMI
Co-Founder, CEO, and President
If your health plan is doing a prospective HEDIS® review (aka prospective HEDIS® or concurrent HEDIS®), you are very likely using some type of software platform, possibly supported by natural language processing (NLP). NLP is an important tool in moving to prospective HEDIS® because it helps reduce the human effort needed to process such a large volume of charts. The value of your prospective HEDIS® process will be directly proportional to how much of your population you can cover.
Let’s assume you’re using a platform similar to Astrata’s Chart Review for prospective HEDIS® — how do you optimize your review? These ten tips and tricks will help you derive maximal value:
1. Select your Measures and Sub-Measures Carefully. Start with the assumption that each measure differs in the data needed to reach an accurate rate. In some cases, you are better served by using unstructured data and NLP; in other cases, you’re better served by structured data sources, for example through CCDs. Carefully examine where you’re getting a lift from MRR and focus on those measures. Be strategic in how you choose measures, and agile in how you change your focus measures from year to year.
2. Manage your HEDIS® Engine Turnover. Your ability to drive prospective review depends on your ability to identify gaps during the measurement year, and that in turn will likely depend on your HEDIS® engine vendor, and/or other processes you use to create a prospective gap list before your engine turnover. If you can push your vendor to turn over your HEDIS® engine earlier in the year, you’ve gained an important advantage for maximizing your time to review. Alternatively, you can use other processes to approximate a gap list for the measurement year. The key is to get that list as early in the year as possible.
3. Get Your Code Alignment in Shape. Ultimately, your review will be only as good as your pseudoclaims. To make sure all that hard abstraction work counts, every closure reason must map to an appropriate gap closure code in your HEDIS® engine. And of course, these can change from year to year. Updating pseudoclaim codes can be a laborious, time-intensive process. But tooling can help – check out the cool new tools in Astrata’s Chart Review that streamline the pseudo-claim code entry and approval process to ensure you get full credit.
4. Automate as Much as You Can. Most HEDIS® analytics teams operate with a mix of scripting and brute-force human effort. This can involve brittle, error-prone, Excel-supported workflow tooling, which costs time and money. If you can create a fully automated loop such that humans are only required to sign off on the closeable gaps, you’ll reduce the effort and cost to your HEDIS® analytics team.
5. Measure NLP Accuracy. Prospective HEDIS® is a game of space and time. You’ve got to cover a lot of space (number of gaps) in a short amount of time. That means you need high-accuracy NLP to minimize the number of non-compliant charts your team has to read, and also to bring them to the HEDIS® evidence as quickly as possible for compliant charts. It’s important to understand how NLP accuracy is measured and to insist on best-practice evaluation processes from your vendors.
6. Reduce the Clicks for Abstractors. Even with high accuracy, your results will depend on how quickly your abstractors can review charts. Look for ways to optimize your chart review workflow. Your review software should move you swiftly to the right place, without a lot of scrolling, and minimize the number of clicks required for abstractors and over-readers to close a gap.
7. Keep Moving Backward in Time. Take a longer-term view of the process. In your first year, you might start a prospective review in September and limit your review to what you can accomplish before your team needs to switch back to a hybrid review in January. But in your second year, you can move up to a July or August start date and increase the time available for prospective review by one month. Ultimately, you’ll keep moving that time backward as the hybrid sample disappears, until you achieve a year-round process that starts in April – or as soon as NCQA releases measures and value sets for the measurement year.
8. Help Your Abstractors Shift Mindset. One of the biggest challenges you’ll face is the psychological shift abstractors experience when they go from doing a hybrid sample review to a full prospective review. Hybrid sample season requires a deep inspection of a finite set of thousands of charts. Each one is precious. In contrast, the prospective review is a bottomless list of gaps to close, where the goal is to close as many as possible in the time allotted. In our experience, discussing this change explicitly during training helps MRR teams quickly adapt and shift their mindset.
9. Leverage Leads as well as Hits. Another place where prospective review adds value is in finding groups of members who do not precisely meet the NCQA requirements for compliance, but may reflect imperfect documentation or could be compliant with minimal additional effort. These cases, which many groups call “leads”, can also be managed during the prospective process, and can contribute to additional rate improvements. Make sure you include lead management in your approach.
10. Measure Impact. Measuring the value of your year-round process is the first step in increasing value. Your HEDIS® engine vendor likely has a report that determines the independent value of each unique source of supplemental data, when given some “priority” of the data sources. This is a valuable tool for measuring the impact of your prospective HEDIS® process when compared to other data sources. Remember that the value is likely to vary depending on measures and lines of business, so be sure to assess value separately across segments. This will help you make better decisions for the following year.
If you’re interested in seeing how Astrata’s purpose-built chart abstraction tools help HEDIS® medical record review teams implement a state-of-the-art review process, contact us for a demonstration, or follow us on LinkedIn.